Contents
Hospital IP Revenues
Successful hospitals get up to 65% of their revenue from approximately 18% of their patients. Most of this is coming from IPD services. Imagine a hospital losing 5% of these high value patients to competition. Would not that lead to loss of up to 20% of total hospital revenue? I am sure hospitals must have thought about this but feel helpless on how to identify these leaked patients in real time.Challenges in Extracting Leakages in IP Admissions
Every hospital is keen, whether successful or otherwise, to know leakages in IP Admissions. But there are challenges in getting that information. Many hospitals try to get the admission request forms filled up to capture these patients. But I see following issues with this approach:- Request forms are usually on paper and are hardly digitized to measure leakages. Even if done, it would be an isolated study and not real-time.
- This form is only filled when patients agrees with Doctor to get admitted. What about patients who never reached the stage of getting request form filled? This would be a large number whose information is lost.
Solution: AI Based Real Time IP Admission Prediction
Artificial Intelligence is very promising approach to solve some of the critical problems in health care. We have used AI to come up with proprietary technology which can predict OP patients who are at risk of getting admitted in near future but have not been admitted yet. This can be used to provide better quality care to patients and ensure that quality care reaches patients pro-actively.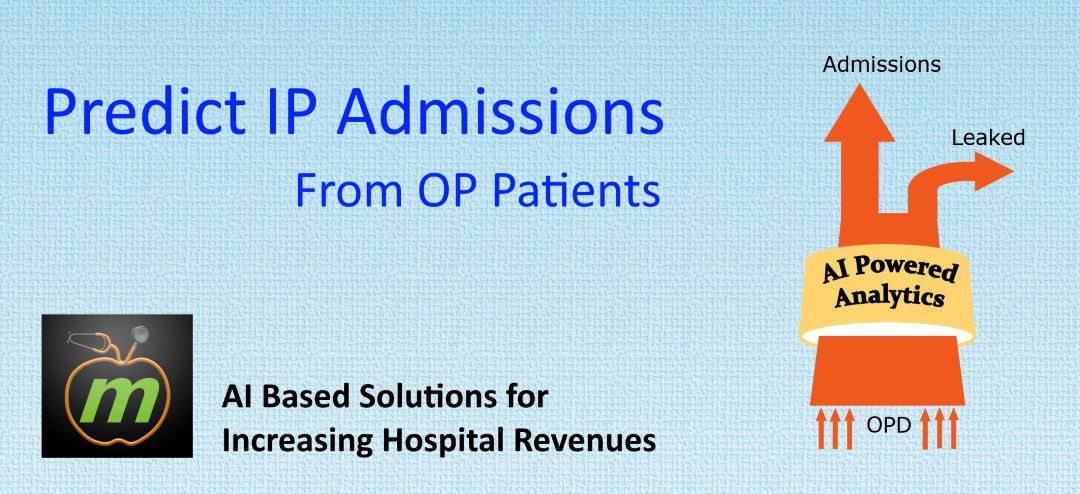
How It Works?
The system reads clinical data of existing patients to learn what kind of patients with certain clinical history get admitted and learn from it. Challenge however is the vast size of input vector size (for example due to different branded drugs at different locations). The algorithm abstracts the clinical data to compress the vector size which works well even on smaller training data sets. The trained model screens OP patients daily and predicts patients with high risk of admission. The models are flexible with real time risk tuning to filter out patients. For example you can use filters to dump out only top 5% of patients with highest risk. The tools also provides interface to browse through complete history of patient. This tool is integrated with CRM tools for an improved engagement with patient and retention.Summary
Aggregate Rating
5 based on votes
Brand Name
mTatva
Product Name
Health-PIE